EXPERIMENT AND RESEARCH ON PREDICTION MODEL OF FOREST FIRE SPREAD BASED ON ENSEMBLE KALMAN FILTER
Abstract
The spread of forest fire is an extremely complex and harmful natural phenomenon. At present, the forest fire spread model has some shortcomings, such as complex formula, inaccurate simulation value and so on. In this paper, the Ensemble Kalman Filter(ENKF) algorithm is applied to the field of forest fire spread so that it can better predict the spread of forest fire. Firstly, the Rothermel forest fire speed formula is simplified, and the simplified Rothermel speed value is modified by the actual measured forest fire spread speed value, so that the optimal model simulation value is obtained. Then the optimal speed is input into Cellular Automata(CA) to simulate the spread of forest fire. Secondly, the experiment is carried out by changing the slope, bed thickness, moisture content, load and wind speed. And the actual measured speed value, the simplified Rothermel model value and the optimized value after ENKF are compared in the process of fire spread. Finally, The experimental results show that the error of fire spread speed corrected by ENKF is smaller, the forest fire spread contour obtained from the optimal speed value by ENKF is closer to the actual fire spread contour, and the highest similarity index is 0.854. The model proposed in this paper has the ability to predict the spread of forest fire indoors.
Keywords
Full Text:
PDFReferences
Dhall A. , Dhasade A. , Nalwade A. , et al. 2020. A survey on systematic approaches in managing forest fires. Applied Geography, 121:102266. DOI:10.1016/j.apgeog. 2020.102266.
Denham M. , Wendt K. , Bianchini G. , et al. 2012. Dynamic Data-Driven Genetic Algorithm for forest fire spread prediction. Journal of Computational Science, 3(5):398-404. DOI:10.1016/j.jocs.2012.06.002 .
Finney M. A. , Grenfell I. C. , Mchugh, C. W. , et al. 2011. A method for ensemble wildland fire simulation. ENVIRONMENTAL MODELING AND ASSESSMENT, 16(2): 153-167. DOI:10.1007/s10666-010-9241-3.
Finney M. , Cohen J. , McAllister S. , Jolly W. 2013. On the need for a theory of wildland fire spread. International Journal of Wildland Fire, 22(1): 25–36. Doi:10.1071/WF11117.
Hua J. , Zhang S. , Gao H. , et al. 2020. Optimizing the Rothermel model for easily Predicting spread rate of forest fire. Mathematical and Computational Forestry and Natural-Resource Sciences, 12(2): 62-71. DIO:
Hui S , Rui X , Li Y. , et al. 2016. An Improved Forest Fire Spread Simulation Algorithm Coupled with Cellular Automata. Geomatics and information Scicnce of Wuhan University, 41(10):1326-1332. DOI:10. 13203/j. whugis20140811.
Jin X. , Kumar L. , Li Z. , et al. 2018. A review of data assimilation of remote sensing and crop models. European Journal of Agronomy, 92:141-152. DOI:doi.org/10.1016/j.eja.2017.11.002.
Metref, S. , Hannart, A. , Ruiz, J, et al. 2019. Estimating model evidence using ensemble-based data assimilation with localization - the model selection problem. Quarterly Journal of the Royal Meteorological Society, 145:1571-1588. DOI:10.1002/qj.3513.
Ntinas V., Moutafis B. , Trunfio G. , et al. 2016. Parallel Fuzzy Cellular Automata for Data-Driven Simulation of Wildfire Spreading. Journal of Computationalence, 21(7):469-485. DOI:10.1016/j.jocs.2016.08.003.
Pagnini, G. , Mentrelli, A. . 2014. Modelling wildland fire propagation by tracking random fronts. Natural Hazards and Earth System Sciences, 14(6): 6521-6557. DOI:10.5194/nhess-14-2249-2014.
Pilo G. , Oke P. , Richard C. , et al. 2018. Impact of data assimilation on vertical velocities in an eddy resolving ocean model. Ocean Modelling, 131:71-85. DOI:10.1016/j.ocemod.2018.09.003.
Rossi L. , Molinier T. , Akhloufi M. . 2019. Advanced stereovision system for fire spreading study . Fire Safety Journal. 60(8):64-72. DOI:10.1016/j.firesaf.2012.10.015.
Reichle, R. H., Walker, J. P ., Koster, R. D., et al. 2002. Extended versus Ensemble Kalman filtering for land dataassimilation.Hydrometeorol, 3:728–740. DIO: 10.1175/1525-7541(2002)003<0728:EVEKFF>2.0.CO;2.
Rochoux M., Emery C. , Ricci S. , et al. 2014. Towards predictive data-driven simulations of wildfire spread - Part II: Ensemble Kalman Filter for the state estimation of a front-tracking simulator of wildfire spread. Natural Hazards and Earth System Sciences Discussions, 2(5):3769-3820. DOI:10.5194/nhess-15-1721-2015.
Sun T. , Zhang L. , Chen W. , et al. 2013. Mountains forest fire spread simulator based on geo-cellular automaton combined with wang zhengfei velocity model. IEEE Journal of Selected Topics in Applied Earth Observations and Remote Sensing, 6(4): 1971-1987. DOI: 10.1109/JSTARS.2012.2231956.
Tartés Cencerrado A. , A Cortés, Margalef . 2014. Towards a dynamic data driven wildfire behavior prediction system at european level. Procedia Computer Science, 29: 1216-1226. DOI:10.1016/j.procs.2014.05.109.
Valero M. , Rios O. , Mata C. , et al. 2017. An integrated approach for tactical monitoring and data-driven spread forecasting of wildfires. Fire Safety Journal, 91(7):835-844. DOI:10.1016/j.firesaf.2017.03.085.
Zhou, T. , Ding, L. , Ji J., et al. 2020. Combined estimation of fire perimeters and fuel adjustment factors in farsite for forecasting wildland fire propagation. Fire Safety Journal, 116(9):103167. DOI: doi.org/10.1016/j.firesaf.2020.103167.
Refbacks
- There are currently no refbacks.

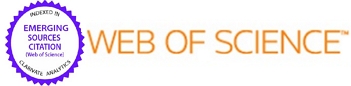
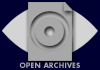
© 2008 Mathematical and Computational Forestry & Natural-Resource Sciences