Evaluating different models to predict biomass increment from multi-temporal lidar sampling and remeasured field inventory data in south-central Alaska
Abstract
We evaluated two sets of equations for their predictive abilities for estimating biomass increment using successively acquired airborne lidar and ground data collected on western lowlands of the Kenai Peninsula in south-central Alaska. The first set included three base equations for estimating biomass increment as a function of lidar metrics, and the remaining equations enhanced the three base equations by considering the hierarchical structure of the data.
It is shown that the mixed effect framework substantially improved the accuracy and precision of biomass increment prediction over the fixed effects that assume the observations are independent for the area covered by two lidar acquisitions, 5 years apart from one another. On the average, root mean square error values were reduced by 19.8% by using a plot-level random coefficient model that account for the impacts of site (biophysical factors) on biomass increment on the western Kenai Peninsula. Â
Mixed effect models are effective statistical tools, but their effective application requires some sample growth data. As such, we recommend two models for estimating biomass increment on the Kenai Peninsula. Â If a subsample of ground data is available to predict the plot random intercept, the enhanced model is suggested. Â
Keywords
Full Text:
PDFReferences
Andersen, H.-E., R.J. McGaughey, and S.E. Reutebuch. 2005. Estimating forest canopy fuel parameters using LIDAR data. Remote Sensing of Environment. 94: 441-445.
Andersen, H. E., T. Clarkin, K. Winterberger, and J. Strunk. 2009. An accuracy assessment of positions obtained using survey- and recreational-grade global positioning system receivers across a range of forest conditions within the Tanana Valley of interior Alaska. Western Journal of Applied Forestry. 24: 128-136
Andersen, H.-E., J. Strunk, and H. Temesgen. 2011. Using airborne lidar as a sampling tool for estimating forest biomass resources in the upper Tanana Valley of interior Alaska. Western Journal of Applied Forestry. 109: 371-377.
Baker, J.S., B.A. McCarl, B.C. Murray, S.K. Rose, R.J. Alig, D. Adams, G. Latta, R. Beach, and A. Daigneault. 2010. Net farm income and land use under a U.S. greenhouse gas cap and trade. Policy Issues (P. 17) 7:1-5
Balzter, H., L. Skinner, A. Luckman, and R. Brooke. 2003. Estimation of tree growth in a conifer plantation over 19 years from multi-satellite L-band SAR. Remote Sensing of Environment. 84: 184–191.
Bollandsås, O. M. and E. Naesset. 2010. Using delta values of multi-temporal first-return small footprint airborne laser scanner data to predict change of tree biomass in mountain spruce forests. Silvilaser 2010 proceedings, September 14th - 17th, Freiburg, Germany.
Bollandsås, O. M., T. Gregoire, E. Næsset, and B. H. Øyen, 2013.Detection of biomass change in a Norwegian mountain forest area using small footprint airborne laser scanner data. Statistical Methods & Applications. 22: 113-129
Breidenbach, J., R.J. McGaughey, H.-E. Andersen, G. Kändler, and S.E. Reutebach. 2007. A mixed effects model to estimate stand volume by means of small footprint airborne lidar data for an American and a German study site. In: Rönnholm, P., Hyyppä, H. and Hyyppä, J. (eds.). Proceedings of ISPRS Workshop Laser Scanning 2007 and Silvilaser 2007, September 12–14, 2007, Finland. International Archives of Photogrammetry, Remote Sensing and Spatial Information Sciences XXXVI(Part 3/W52). p. 77–83.
Breidenbach, J., E. Kublin, R. McGaughey, H.-E. Andersen, and S.E. Reutebuch. 2008. Mixed-effects models for estimating stand volume by means of small footprint airborne laser scanner data. Photogrammetric Journal of Finland. 21: 4-15.
Cairns, M.A., I. Olmsted, J. Granados, and J. Argaez. 2003. Composition and aboveground tree biomass of a dry semi-evergreen forest on Mexico’s Yucatan Peninsula. For. Ecol. and Mgmt. 186: 125-132.
Carroll, R.J., D. Ruppert, L.A. Stefanski, and C. Crainiceanu. 2006. Measurement error in nonlinear models. A modern perspective, 2nd ed. Chapman and Hall.
Diggle, P., P. Heagerty, K.Y. Liang, and S. Zeger. 2002. Analysis of longitudinal data. 2nd ed. Oxford Press, Oxford.
Donoghue, D.N.M., P.J. Watt, N.J. Cox, R.W. Dunford, J. Wilson, S. Stables, and S. Smith. 2004. An evaluation of the use of satellite data for monitoring early development of young Sitka spruce plantation forest growth. Forestry. 77: 383–396.
Edmons, R.L., K.K. Agee, and R.I. Gara. 2000. Forest health and protection. McGraw-Hill, 630 pp.
Fitzmaurice, G., M. Davidian, G. Molenberghs, and G. Verbeke. 2008. Longitudinal data analysis. Boca Raton, Florida, Chapman & Hall/CRC.
Goerndt, M.E., V.J. Monleon, and H. Temesgen. 2010. Relating forest attributes with area- and tree-based Lidar metrics for western Oregon. Western Journal of Applied Forestry. 25: 105-111.
Gough, C.M., C.S. Vogel, H.P. Schmid, and P.S. Curtis. 2008. Controls on annual carbon storage: lessons from the past and predictions for the future. BioScience. 58:7:609-622.
Goldfield, S.M. and R.E. Quandt. 1965. Some tests for homoscedasticity. J. Amer. Stat. Assn. 60: 539-547.
Gregoire, T. G. 1987. Generalized error structure for forestry yield models. Forest Science. 33: 423-444.
Hall, D.B. and R.L. Bailey. 2001. Modeling and prediction of forest growth variables based on multilevel nonlinear mixed models. Forest Science. 47: 311–321.
Hazard, J.H., H. Schreuder, and V. LaBau. 1995. The Alaska four-phase forest inventory sampling design using remote sensing and ground sampling. Photogrammetric Engineering and Remote Sensing. 61(3): 291 - 302.
Hudak, A. T., E. K. Strand, L. A. Vierling, J.C. Byrne, J. U. H Eitel,.S. Martinuzzi, and M.J. Falkowski. 2012. Quantifying aboveground forest carbon pools and fluxes from repeat LiDAR surveys. Remote Sensing of Environment. 123:25-40.
Hyde, P., R. Dubayaha, W. Walker, J. Bryan Blair, M. Hofton and C. Hunsaker. 2006. Mapping forest structure for wildlife habitat analysis using multi-sensor (Lidar, SAR/InSAR, ETM+, Quickbird). Remote Sensing of Environment. 120: 63-73.
Lohr, S.L. 1999. Sampling: Design and Analysis. Duxbury, Pacific Grove, CA. 494 p.
Ming, S.X. and S. Huang. 2010. Incorporating correlated error structures into mixed forest growth models: prediction and inference implications. Can. J. For. Res. 40(5): 977-990.
Monleon, V.J. 2003. A hierarchical linear model for tree height prediction. In: Proceedings of the 2003 meeting of the American Statistical Association, Section on Statistics and the Environment. Alexandria, VA p. 2865-2869.
Meyer, V. S.S. Saatchi, J.Chave, J.W.Dalling, S.Bohlman, G.A.Fricker, C.Robinson, M.Neumann, and S.Hubbell, 2013. Detecting tropical forest biomass dynamics from repeated airborne lidar measurements. Biogeosciences. 10: 5421-5438.
McRoberts, R.E., O. M. Bollandsås, and E. Næsset. 2014. Modeling and estimating change. In. M. Maltamo et al. (eds.), Forestry Applications of Airborne Laser Scanning: Concepts and Case Studies. Managing Forest Ecosystems. Springer. Pp. 269-293.
Næsset, E., O. M. Bollandsås, T. Gobakken, T. Gregoire, and G. Ståhl. 2013. Model-assisted estimation of change in forest biomass over an 11 year period in a sample survey supported by airborne LiDAR: A case study with post-stratification to provide "activity data". Remote Sensing of Environment. 128: 299-314.
Næsset, E., T. Gobakken, J. Holmgren, H. Hyyppä, J. Hyyppä, and M. Maltamo 2004. Laser scanning of forest resources: The Nordic experience. Scandinavian Journal of Forest Research. 19: 482–499
Næsset, E. and T. Gobakken. 2005. Estimating forest growth using canopy metrics derived from airborne laser scanner data. Remote Sensing of Environment. 96: 453–465.
Neter, J., W. Wasserman, and M.H. Kutner. 1990. Applied linear regression models. Richard D. Irwin. Boston. 1181 pp.
Pinheiro, J.C. and D. M. Bates. 2000. Mixed effects models in S and S-plus. Springer, New York, 528 pp.
R Development Core Team. 2011. R: a language and environment for statistical computing. R Foundation for Statistical Computing, Vienna, Austria. Available at http://www.R-project.org.
Roesch, F.A. and G.A. Reams. 1999. Analytical alternatives for an annual inventory system. Journal of Forestry. 97: 44-48.
Ståhl, G., H. Sören, T. Gregoire, T. Gobakken, E. Næsset, and R. Nelson.2011. Model-based inference for biomass estimation in a LiDAR sample survey in Hedmark County, Norway. Can. J. For. Res. 41: 96-107.
Temesgen, H., V.J. Monleon, and D.W. Hann. 2008. Analysis of nonlinear tree height prediction strategies for Douglas-fir forests. Can. J. For. Res. 38: 553-565.
Temesgen, H., M.E. Goerndt, G. P. Johnson, D.M. Adams, and R.A. Monserud. 2007. Forest measurement and biometrics in forest management: status and future needs of the Pacific Northwest USA. Journal of Forestry. 105: 233-238.
Yarie, J., E. Kane, and M. Mack. 2007. Aboveground biomass equations for the trees of interior Alaska. Agricultural and Forestry Experiment Station Bulletin: 115. University of Alaska-Fairbanks. 15 p.
Yu, X., J. Hyyppa, H. Kaartinen, and M. Maltamo. 2004. Automatic detection of harvested trees and determination of forest growth using airborne laser scanning. Remote Sensing of Environment. 90: 451–462.
Yu, X., J. Hyyppa, A. Kukko, M. Maltamo, and H. Kaartinen. 2006. Change detection techniques for canopy height growth measurements using airborne laser scanner data. Photogrammetric Engineering and Remote Sensing. 72: 1339–1348.
van Hees, W.W.S. 2005. Spruce reproduction dynamics on Alaska’s Kenai Peninsula, 1987-2000. United States Department of Agriculture Forest Service Pacific Northwest Research Station Research Paper PNW-RP-563.
Refbacks
- There are currently no refbacks.

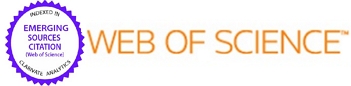
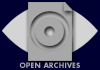
© 2008 Mathematical and Computational Forestry & Natural-Resource Sciences