Comparing spatial and non-spatial approaches for predicting forest soil organic carbon at unsampled locations
Abstract
Prediction of soil organic carbon (SOC) at unsampled locations is central to statistical modeling of regional SOC stocks. This is often accomplished by applying geostatistical techniques to plot inventory data. However, in many cases inventory data is sparsely sampled (<0.1 plots/km^2) relative to the region of interest, and it is unknown if geostatistics provides any advantage. Our objective was to test whether modeling spatial autocorrelation, in multivariate and univariate predictive models, improved estimates of SOC at prediction locations based on sparsely-sampled inventory data. We conducted our study using a dataset sampled across all forested land in the Coastal Plain physiographic province of New Jersey, USA. We considered five models for predicting SOC, two linear regression models (intercept only and multiple regression with predictor variables), ordinary kriging (a univariate spatial approach), and two multivariate spatial methods (regression kriging and co-kriging). We conducted a simulation study in which we compared the predictive performance (in terms of root mean squared error) of all five models. Our results suggest that our sparsely-sampled SOC data exhibits no spatial structure (Moran’s I=0.05, p=0.39), though several of the covariates are spatially autocorrelated. Multiple linear regression had the best performance in the simulation study, while co-kriging performed the worst. Our results suggest that when inventory data is dispersed across the region of interest, modeling spatial autocorrelation does not provide significant advantage for predicting SOC at unsampled locations. However, it is unknown whether this autocorrelation does not exist at broad scales, or if sparse sampling strategies are unable to detect it. We conclude that in these situations, multiple regression provides a straightforward alternative to predicting SOC for mapping studies, but that more work on the spatial structure of soil carbon across multiple scales is needed.
Keywords
Forest soil carbon; Geostatistics; Regression; Co-kriging; New Jersey; Coastal Plain
Refbacks
- There are currently no refbacks.

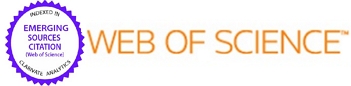
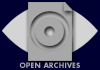
© 2008 Mathematical and Computational Forestry & Natural-Resource Sciences